Virtualization 90 Minute Demonstration Crash Free udemy Cour
Virtualization 90 Minute Demonstration Crash Free udemy Course
Welcome to the world of virtualization ! In this comprehensive crash course, we’ll unravel the complexities of virtualization technologies, empowering you with the knowledge you need to navigate VMware vSphere, Microsoft Hyper-V, and AWS with confidence. Whether you’re a beginner or seeking a refresher, this course is tailored to demystify virtualization concepts in just 90 minutes.
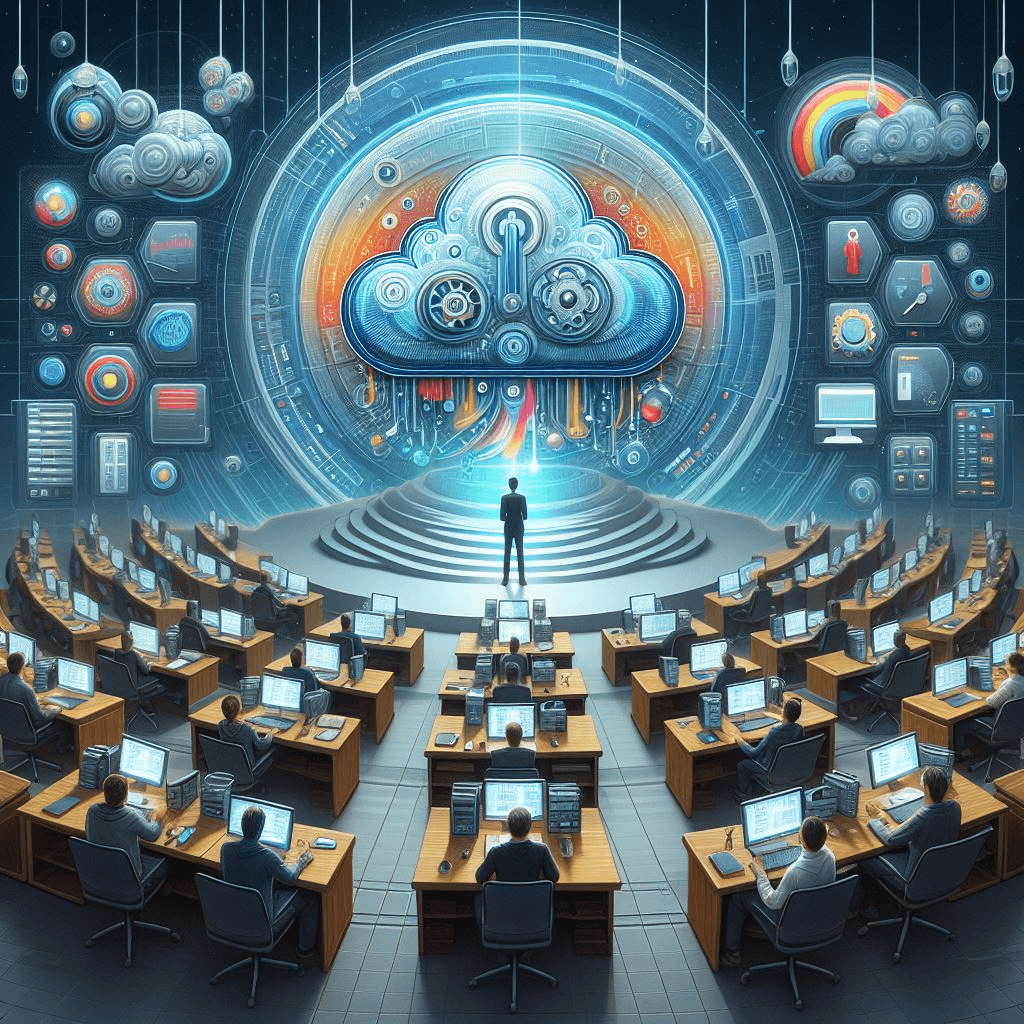
Understanding Virtualization Basics
In this section, we’ll dive into the fundamental concepts of virtualization. From defining VMs, Hosts, and Hypervisors to understanding the shared resources of a host among multiple VMs, you’ll gain a solid foundation in virtualization essentials. We’ll strip away the technical jargon and focus on clear, concise explanations to ensure comprehension.
Types of Hypervisors: Unveiling the Key Differences
Delve into the world of Hypervisors in this segment. We’ll distinguish between Type-1 and Type-2 Hypervisors, exploring their unique characteristics and functionalities. By understanding the nuances of each type, you’ll be equipped to make informed decisions when choosing virtualization solutions.
Mastering VM Configuration and Management
Unlock the secrets behind VM configuration and management in this hands-on section. Learn how VM files are structured and gain insights into the live state of a VM. Discover how CPU, memory, network, and storage resources are allocated to VMs, laying the groundwork for efficient VM deployment and operation.
https://kalinkainfotech.com/category/blog/udemy-courses
Exploring the Benefits of Virtualization
In this segment, we’ll delve into the myriad benefits of virtualization. From enhanced mobility and resource efficiency to improved availability and scalability, you’ll discover how virtualization revolutionizes IT infrastructure. Gain a holistic understanding of the advantages that virtualization brings to organizations of all sizes.
Hands-On Practice: Creating VMs
Get ready to roll up your sleeves and put theory into practice! We’ll guide you through the process of creating VMs on your local machine using VMware Workstation Player. Additionally, you’ll learn how to leverage the AWS Free Tier to deploy VMs in the Cloud, gaining practical experience in VM creation and management.
Conclusion: Your Gateway to Virtualization Mastery
As we conclude our crash course, you’ll emerge with a newfound understanding of virtualization fundamentals. Whether you’re embarking on a career in IT or seeking to expand your knowledge, this course serves as your gateway to virtualization mastery. With clear explanations, hands-on practice, and real-world applications, you’ll be well-prepared to tackle VMware, Hyper-V, or cloud-based virtualization with confidence.
Virtualization 90 Minute Demonstration Crash Free udemy Cour
the secrets of virtualization in just 90 minutes with comprehensive course! practical skills real-world applications for VMware vSphere, Microsoft Hyper-V, AWS.
Who Should Take This Course?
This course is perfect for anyone eager to grasp the basics of virtualization for VMware, Hyper-V, or the cloud. Whether you’re a novice or seeking a refresher, our beginner-friendly approach ensures that learners of all backgrounds can benefit from this crash course.