AWS Zero to Hero: A Comprehensive Guide for Beginners Course
AWS Zero to Hero: A Comprehensive Guide for Beginners
Amazon Web Services ( AWS ) has become an integral part of the modern tech landscape, providing a robust and scalable cloud computing platform. The ” AWS Zero to Hero” course is designed for beginners, offering a hands-on approach to mastering essential AWS services. This comprehensive course covers key functionalities such as AWS EC2, RDS, S3, SES, and CloudWatch, making it an ideal starting point for anyone eager to dive into the world of cloud computing.
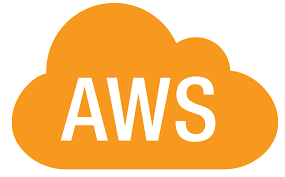
Course Overview:
- Launch an AWS EC2 WordPress Web Server:
In the first module, participants will learn how to set up a fully functional WordPress web server on AWS EC2. The course provides step-by-step guidance on creating, configuring, and launching an EC2 instance, making it accessible to beginners with no prior AWS experience. - Create and Connect to an AWS RDS Relational Database Server:
Building on the foundation of the EC2 module, the course guides learners through the process of launching and connecting to an AWS RDS relational database server. Participants will gain practical insights into database management and connectivity within the AWS ecosystem. - Create a Highly Available and Fault-Tolerant NodeJS Environment:
For those interested in application development, the course explores the creation of a highly available and fault-tolerant environment for NodeJS applications using AWS Elastic Beanstalk. This module emphasizes best practices for ensuring the reliability of applications in a cloud environment. - Upload Files to AWS S3:
AWS Simple Storage Service (S3) is a fundamental storage solution in the AWS portfolio. Participants will learn how to store and retrieve files from S3, gaining a practical understanding of object storage and its applications. - Send Email with AWS SES:
Communication is key in any application. The course delves into the Amazon Simple Email Service (SES), demonstrating how to send emails seamlessly within AWS. Participants will grasp the essentials of email integration in cloud-based applications. - Monitor and Alert with AWS CloudWatch:
Monitoring and alerting are crucial aspects of maintaining a healthy cloud infrastructure. The course introduces AWS CloudWatch, guiding participants through the creation of alarms and the monitoring of various AWS resources to ensure optimal performance. - How to Use AWS for Free:
Cost management is an essential skill for AWS users. The course wraps up by providing insights into how participants can utilize AWS services for free, making it accessible for those on a budget.
Conclusion:
The “AWS Zero to Hero” course is a high-paced, hands-on learning experience that empowers beginners to navigate the AWS landscape confidently. With practical examples and professional course notes, participants gain valuable skills in deploying web servers, managing databases, developing fault-tolerant applications, and more. This course serves as an excellent foundation for those looking to pursue further certifications, such as the AWS Certified Associate. Whether you’re a developer, IT professional, or anyone eager to harness the power of AWS, this course is your gateway to becoming an AWS hero.