Mastering Data: The Best Tech Stacks for Enthusiasts Revealed
Welcome, fellow data enthusiasts! In this exhilarating journey through the realm of data technology, we’ll explore the most potent tools and frameworks that empower you to wield the magic of data. From cloud platforms like AWS and Azure to powerful analytics engines like Hadoop and Spark, there’s a treasure trove of possibilities waiting to be discovered. Whether you’re a seasoned data wizard or just starting your adventure, this guide will illuminate the path to building your dream tech stack.
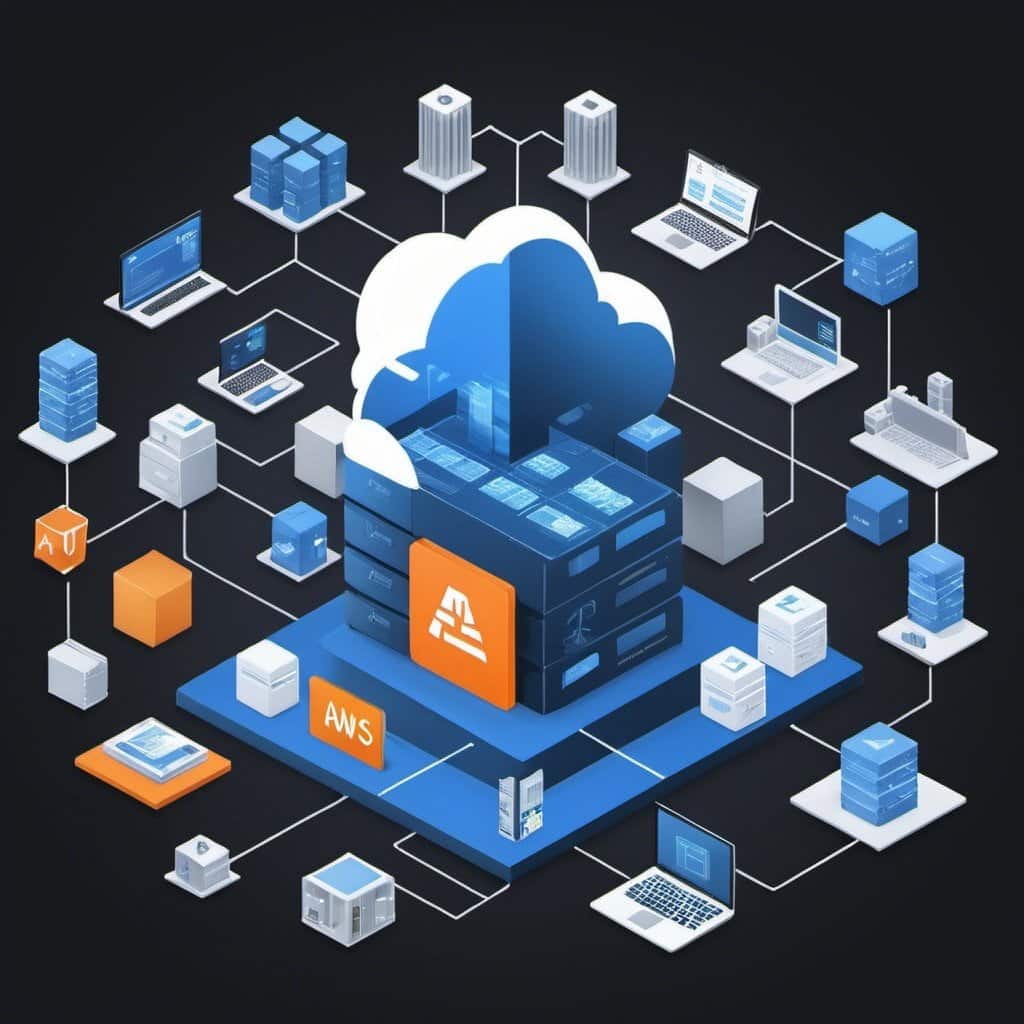
The Powerhouses: AWS and Azure
At the heart of modern data operations lie the cloud giants AWS and Azure. These platforms offer a vast array of services tailored to data processing, storage, and analysis. With AWS’s Redshift and Azure’s data services, you can effortlessly scale your data infrastructure to meet growing demands. Both platforms provide seamless integration with popular analytics tools like Hadoop, Spark, and SQL databases, making them indispensable for any data enthusiast.
Redshift: Unleashing the Power of Data Warehousing
AWS Redshift stands as a beacon of innovation in the realm of data warehousing. With its unparalleled performance and scalability, Redshift empowers organizations to analyze massive datasets with lightning speed. Leveraging columnar storage and advanced query optimization techniques, Redshift ensures rapid insights into your data, transforming raw information into actionable intelligence. Whether you’re crunching numbers or uncovering hidden trends, Redshift serves as the cornerstone of any robust data stack.
Section 3: Hadoop and Spark: The Dynamic Duo of Big Data
When it comes to processing vast amounts of data, few tools rival the prowess of Hadoop and Spark. Hadoop’s distributed file system and MapReduce paradigm revolutionized the field of big data, enabling parallel processing of massive datasets across clusters of commodity hardware. Spark, with its lightning-fast in-memory processing engine, takes big data analytics to new heights, offering real-time insights and interactive querying capabilities. Together, Hadoop and Spark form an unbeatable combination for tackling the toughest data challenges.
Section 4: SQL: The Language of Data Manipulation
SQL, or Structured Query Language, serves as the lingua franca of data manipulation. With its intuitive syntax and powerful querying capabilities, SQL allows you to extract, transform, and analyze data with ease. Whether you’re retrieving records from a database, joining tables, or aggregating results, SQL provides a flexible and efficient means of interacting with your data. From simple SELECT statements to complex data transformations, mastering SQL is essential for any data enthusiast looking to unlock the full potential of their datasets.
Section 5: Excel: The Unsung Hero of Data Analysis
In the world of data analysis, Excel reigns supreme as the go-to tool for spreadsheet manipulation and visualization. With its familiar interface and robust feature set, Excel empowers users to perform a wide range of data tasks, from basic calculations to advanced statistical analysis. Whether you’re creating pivot tables, generating charts, or building complex models, Excel provides a versatile platform for exploring and interpreting your data. With the addition of Power Query and Power Pivot, Excel becomes even more powerful, offering seamless integration with external data sources and advanced data modeling capabilities.
Section 6: Python: The Swiss Army Knife of Data Science
Python has emerged as the de facto language for data science and machine learning, thanks to its simplicity, versatility, and vibrant ecosystem of libraries. From data manipulation and visualization with pandas and Matplotlib to machine learning with scikit-learn and TensorFlow, Python offers a comprehensive toolkit for every stage of the data science pipeline. With its clean syntax and extensive documentation, Python enables data enthusiasts to prototype and deploy sophisticated analytics solutions with ease. Whether you’re a seasoned data scientist or just getting started, Python is an indispensable tool in your data arsenal.
Section 7: Hive: Simplifying Big Data Processing with SQL
Hive, built on top of Hadoop, brings the power of SQL to big data processing. By abstracting away the complexities of MapReduce and providing a familiar SQL interface, Hive makes it easy to query and analyze large datasets stored in Hadoop Distributed File System (HDFS). With its rich ecosystem of UDFs (User-Defined Functions) and integration with popular BI tools, Hive enables data enthusiasts to unlock the potential of their big data infrastructure without needing to learn complex programming paradigms. Whether you’re running ad-hoc queries or building data pipelines, Hive simplifies big data processing and empowers you to extract valuable insights from your data.
Section 8: Crafting Your Dream Tech Stack
Now that we’ve explored the essential components of a data tech stack, it’s time to craft your own masterpiece. Whether you prefer the scalability of cloud platforms like AWS and Azure, the power of analytics engines like Hadoop and Spark, or the simplicity of tools like Excel and Python, there’s a perfect combination waiting for you. Experiment, iterate, and find the stack that best suits your needs and preferences. Remember, the journey to becoming a data wizard is as much about exploration as it is about mastery.
Section 9: Conclusion: Embrace the Magic of Data
As we conclude our expedition through the world of data technology, remember that the magic of data lies not just in the tools we use, but in the insights we uncover and the impact we make. Whether you’re analyzing customer behavior, optimizing business processes, or driving innovation, data has the power to transform industries and shape the future. So embrace the magic of data, and let your curiosity and creativity guide you on your quest for knowledge and discovery.