Maximize Your Data Potential with AWS Data Engineering Labs
Unleash Your Data Potential: Exploring AWS Data Engineering Labs
In today’s data-driven world, harnessing the power of data engineering is essential for unlocking insights and driving business success. Amazon Web Services (AWS) offers a rich ecosystem of tools and services tailored for data engineering tasks, empowering organizations to process, analyze, and derive value from their data at scale. In this blog post, we’ll dive into the world of AWS Data Engineering Labs, where data enthusiasts and professionals alike can embark on a journey of discovery, experimentation, and innovation. From data ingestion to transformation, modeling to visualization, AWS provides a playground for honing your data engineering skills and mastering the art of data-driven decision-making.
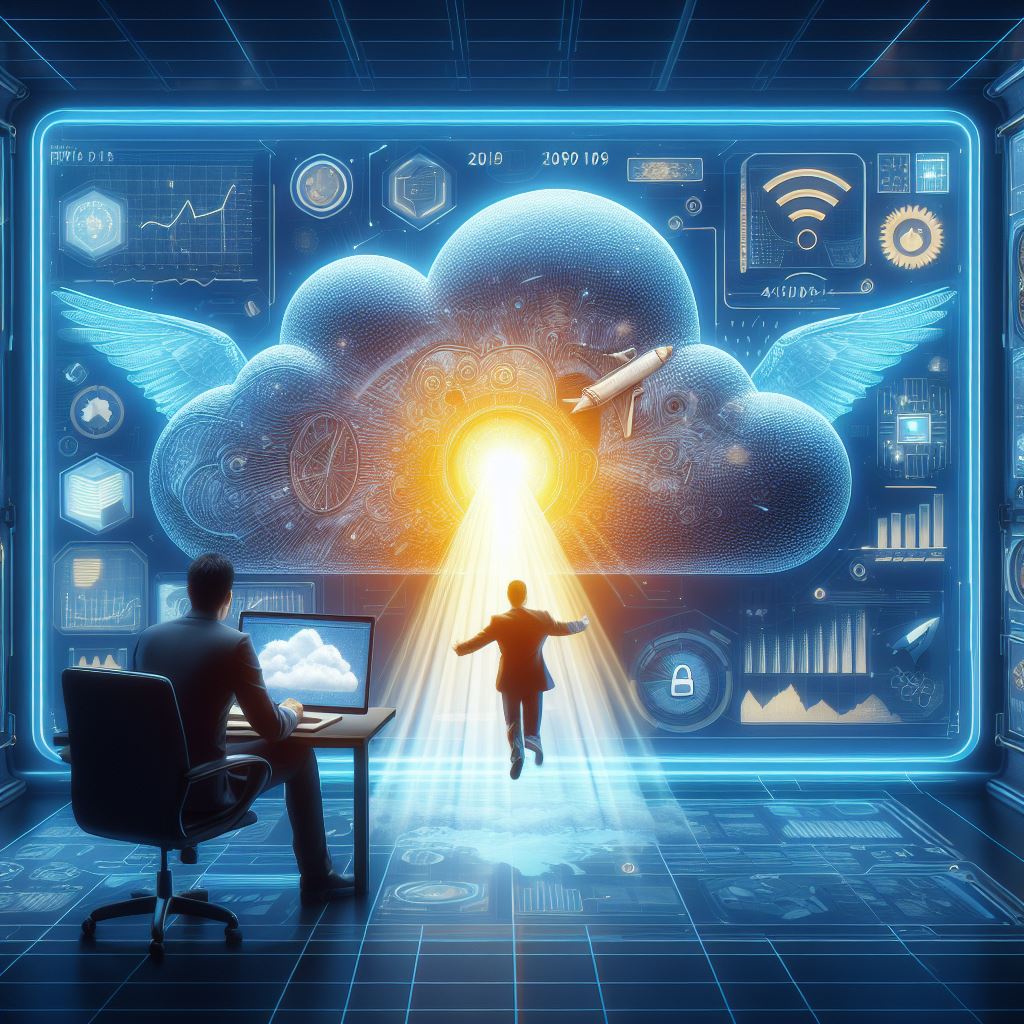
1. Getting Started: Navigating the AWS Data Landscape
Before delving into the depths of AWS Data Engineering Labs, it’s essential to understand the rich tapestry of AWS data services available. From foundational services like Amazon S3 for storage and Amazon RDS for databases to advanced analytics tools like Amazon Redshift and Amazon EMR, AWS offers a comprehensive suite of solutions for every stage of the data lifecycle. By familiarizing yourself with these services, you can effectively navigate the AWS data landscape and choose the right tools for your data engineering needs.
2. Data Ingestion: Bringing Your Data into the Cloud
The journey of data engineering often begins with data ingestion, the process of collecting and importing data into the cloud. AWS provides a myriad of options for data ingestion, ranging from simple file uploads to sophisticated streaming solutions. With services like Amazon Kinesis and AWS Data Pipeline, you can seamlessly ingest data from various sources, including databases, IoT devices, and streaming platforms. Whether you’re dealing with structured or unstructured data, AWS offers the flexibility and scalability you need to ingest data efficiently and reliably.
3. Data Transformation: Shaping Your Data for Analysis
Once your data is securely stored in the cloud, the next step is data transformation, where raw data is refined, cleansed, and prepared for analysis. AWS offers a plethora of tools for data transformation, including Amazon Glue, AWS Lambda, and Amazon EMR. With Glue, you can easily create and manage ETL (Extract, Transform, Load) jobs without the need for infrastructure provisioning or management. Lambda enables you to run code in response to events, making it ideal for real-time data processing tasks. And with EMR, you can process large-scale data sets using popular frameworks like Apache Spark and Hadoop.
4. Data Modeling: Designing Your Data Architecture
Data modeling is the process of designing the structure and relationships of your data to facilitate efficient querying and analysis. In the world of AWS Data Engineering Labs, data modeling is made easy with services like Amazon DynamoDB, Amazon Redshift, and Amazon Aurora. DynamoDB offers a fully managed NoSQL database service, perfect for high-performance, low-latency applications. Redshift, on the other hand, is a fully managed data warehouse solution designed for large-scale analytics workloads. And Aurora combines the performance and availability of high-end commercial databases with the simplicity and cost-effectiveness of open-source databases.
5. Data Analysis: Extracting Insights from Your Data
With your data securely stored, transformed, and modeled, it’s time to extract insights that drive business value. AWS provides a suite of analytics tools for data analysis, including Amazon Athena, Amazon QuickSight, and Amazon Elasticsearch Service. Athena allows you to query data stored in S3 using standard SQL syntax, without the need for complex ETL processes. QuickSight enables you to visualize and explore your data through interactive dashboards and reports. And Elasticsearch Service offers a fully managed Elasticsearch service for real-time search and analytics.
6. Data Visualization: Bringing Your Insights to Life
Data visualization is the art of presenting data in a visual format to communicate insights and trends effectively. AWS offers a range of visualization tools, including Amazon QuickSight and Amazon Quicksight Embedded. QuickSight allows you to create interactive dashboards and reports with ease, using data from various sources including Amazon RDS, Redshift, and S3. Quicksight Embedded, on the other hand, enables you to embed analytics directly into your applications, providing a seamless and integrated data visualization experience for your users.
7. Machine Learning: Unleashing the Power of AI
In the era of artificial intelligence (AI), machine learning (ML) is revolutionizing the way we analyze and interpret data. AWS provides a suite of ML services, including Amazon SageMaker, Amazon Comprehend, and Amazon Rekognition. SageMaker is a fully managed service that enables you to build, train, and deploy ML models at scale. Comprehend offers natural language processing (NLP) capabilities for extracting insights from text data. And Rekognition provides image and video analysis for object detection, facial recognition, and more.
8. Real-world Applications: Putting Your Skills to the Test
The true test of your data engineering skills lies in real-world applications, where you can apply your knowledge to solve complex
Virtualization 90 Minute Demonstration Crash Free udemy Cour
the secrets of virtualization in just 90 minutes with comprehensive course! practical skills real-world applications for VMware vSphere, Microsoft Hyper-V, AWS.